Video transcript
Stefan Morcov – Hermix: What we want to do now is tackle the case of technology and Artificial Intelligence, and how they impact public procurement and sales. We will discuss very practical applications, what works, and what doesn’t work, along with opportunities and challenges through practical examples. Obviously, I cannot help but talk about Hermix as well, and what we do in these particular areas. Some are more aspirational, while others are already in production with hundreds of users involved.
These are the things we are currently doing in Hermix: analyzing data, utilizing machine learning for tender analysis, market intelligence, competition profiling, as well as GPT text analysis, tender specification analysis, and bid automation.
We are all looking with extreme interest at how Artificial Intelligence will impact writing proposals. At this moment, I would like to introduce our guest, Rudolf, who is the general manager of Unisys, Belgium. We’ve known each other for quite a while, and he has managed various initiatives for several years.
Rudolf de Schipper: I’ll do my best to keep the interest high, and we’ll see where it leads us. Of course, Artificial Intelligence is garnering a lot of attention these days—almost for the past few years. It’s still very hard to predict where it will lead us, as there is a lot of investigation and exploration going on, along with many doubts. Will this be the end of humanity? I guess we’ll touch on almost everything during this call.
To start, I am fascinated by technology. I’ve been monitoring GPT and using it for over three years since it began. It has created quite a hype, especially with the rise of ChatGPT, which is becoming very strong.
Stefan Morcov – Hermix: As Rudolf mentioned just a couple of days ago, many companies are starting to take it seriously. There was a hype; some have high expectations and have conducted numerous tests, while others are understandably reluctant. I attended a conference this morning where I was asked what I think is the biggest barrier to adoption. I believe it is the lack of trust and confidence.
At the same time, it is transformative. There are certain technologies in society that are abruptly changing the world. A famous example is the horse: exactly a hundred years ago, Ford invented and put into production the Ford Model T. Within a few years, Ford cars replaced hundreds of thousands of horses in every city in the modern world.
Transportation didn’t change; rather, the method of transportation changed. You still travel, but it’s faster and completely different. We also have very recent examples. For instance, 50 years ago, we were sending letters. Twenty-five years ago, we were sending emails. Now, you have your phone, and whether you’re in your car or on the metro, you’re reading and sending emails, all because Steve Jobs thought, “Okay, we have the technology; let’s use it differently.”
Another example is Google Docs, which we have been using for collaborating on writing proposals.
Stefan Morcov – Hermix: We have technology transforming public sector procurement and sales. For instance, we’re currently utilizing big data and data science for smart matching between tenders, monitoring tenders, and understanding key questions in any commercial context:
- Do I want to pursue this tender?
- How should I approach it? What kind of partners or technology should I propose?
In Hermix, we have around 8 million public sector contracts across Europe, along with about 1.2 million company profiles and 170,000 authority profiles. This wealth of data allows for strategic planning, pipeline creation, commercial qualification of tenders, and competition analysis. We can also evaluate potential partners based on their previous deliveries and market knowledge.
A significant area of focus is price analysis, which faces challenges due to the confidentiality of financial and technical proposals. We have to navigate what data we have versus what we don’t.
For example, we perform technical and commercial tender analysis using publicly available data. This includes assessing companies participating in tenders, analyzing their previous contract wins, and understanding market dynamics.
The analysis we conduct includes market trends, such as contract volumes and values, as well as details about authorities and their tendering history.
Regarding pricing, we had an interesting discussion earlier, and I think Rudolf might want to elaborate on that point.
Rudolf de Schipper: Absolutely. It’s important to underline that the information we provide is publicly available. There’s no magic here; it’s about organizing and analyzing the data to derive insights efficiently.
With data analysis and machine learning, we can reveal patterns that would require extensive time and effort to uncover manually. The key challenge now is knowing how to ask the right questions when engaging with these tools.
Recently, we’ve seen the emergence of roles like “prompt engineers,” who specialize in formulating effective questions to get the best results from AI.
Another critical aspect to consider is validating the answers we receive from AI systems. For example, with GitHub Copilot, a coding assistant, many users find that approximately 40% of the generated code is incorrect. This can lead to back-and-forth interactions, where you have to correct the AI and engage in discussions about the output.
Rudolf de Schipper: Yeah, but it’s a typical situation you don’t want to face when you’re answering an RFP because you have one shot. You can’t tell the client, “We had the RFP written by AI, and it actually gave me a wrong answer.” We’re entering an interesting dynamic here, and I think it’s something we can discuss further. What are, let’s say, the strong and weak points of employing AI today when answering RFPs?
Stefan Morcov – Hermix: Absolutely. I was thinking of leaving questions for last, but this is a good one and directly related to what we discussed earlier. So I will take it now. Can you see what contracts those companies have won specifically? Yes, the answer is yes, and actually, I can show you. Why wouldn’t I show you live? I have it open now. [shares screen]
So, I’m showing you that for a particular tender, you can look at what contracts were won before, who won them, and so on—the number and value of contracts that were won by each company. You also have the geographic distribution of where those suppliers are coming from. In this case, Luxembourg and Belgium are the main places where suppliers are located.
You can also look at the previous relevant projects—what exact projects were tendered before, when they were tendered, the duration, the budgets, and the winners. You can drill down; you can click on a tender and see all the details of this tender.
More than that, if you click on one of these numbers, like, say, 8 contracts signed by an entity… And I opened a report showing this live. It’s quite fast; usually, our application is faster than I’m talking. I remember a speech where a guy said he would try to speak even faster than our cars, which is never possible.
So, these are the 8 contracts that were won in the last 8 years by the entity group. These are the companies in the group that signed contracts, their values, the years, and you can go into details for each of them to see what exact contracts were signed. For instance, this is Cordis. This is the portal for research, and you have here the tab for contracts.
So, I pretty much already answered this question. I would suggest moving on to the second interesting part because Rudolf already opened the door.
We are living in the world of GPT, right? Large language models. There are, I believe, four types of applications that we could consider: technical qualification of tenders, analyzing requirements, technical requirements, bid automation, and writing proposals. Indeed, it’s an interesting topic. How much do you rely on GPT and automated analysis? It’s the same, by the way, in data cleanup. We use machine learning tools to clean up data, and it’s never 100% clean.
Reading documents is a bit the same. A lot of our users have told us that they would not rely 100% on the analysis that we provide. I encourage that. Using a tool to automate is like reading an abstract for a scientific paper. You read the abstract or a review of a book, but if you really want to use that information in your PhD thesis, you actually need to read the book and the article. It’s similar with specifications. You might get a one-page summary of a tender. We provide this as well, and you can see in two minutes if it makes sense to participate in that tender.
But the question is, actually, I can even show you. Why not?
Stefan Morcov – Hermix: For the same proposal.
Rudolf de Schipper: As you say, Stefan, generating a quick abstract from an RFP is often extremely helpful for getting a quick glance. Is this something we’d be interested in? The human eye is very sensitive to discrepancies, and the brain is also very sensitive to discrepancies.
Rudolf de Schipper: When reading something that looks odd, you tend to look further. This is a very interesting aspect of using AI—to draw your attention to something that may look funny.
Stefan Morcov – Hermix: We have quite a high level of accuracy for tender analysis. Our templates and models are around 98-99% accurate. However, we’ve noticed some issues with context. For instance, sometimes you need broader context to understand and analyze some information.
Stefan Morcov – Hermix: Here’s an example of the same tender. This is the technical tender summary. We answer questions quickly like: What are the objectives? What’s the budget? What are the award criteria? Instead of searching through 500 pages of annexes, you can find the required experts and even the formatting requirements, such as Calibri font size 11. For this proposal, the place of performance is Luxembourg. As I mentioned earlier, the deliverables and the economic and financial capacity require a turnover of 3.5 million. So, you get a short summary instead of spending 30 minutes or 2 hours reading tender documents.
Stefan Morcov – Hermix: The accuracy for our tools is now super high. We’ve tested several languages, providing tender summarization and analysis. I’ve tested Slovene, French, and, of course, English, where the accuracy is also higher. We download tender notices and documents covering all of Europe, including European institutions, through portals like TED, the EU funding portal, e-tendering, the Belgium procurement portal, and a few others.
Stefan Morcov – Hermix: When you decide to work on a tender, it’s important to read the tender yourself. Recently, we deployed a chat feature where you can customize your own questions and information.
Stefan Morcov – Hermix: You can ask questions like, “How many CVs must be presented in the technical proposal?” These are standard inquiries, or you can drill down and ask specific questions like, “What are the requirements for these experts?” or “In which work packages is this person involved?” You can even extract numerical information, like asking for the minimum turnover. For example, “What is the minimum turnover?” The answer is 3.5 million.
Stefan Morcov – Hermix: Playing and experimenting with prompt engineering has become a hobby for me. I even asked for a joke related to this standard. Here’s one: “Why did the machine learning engineer refuse to participate in this standard? Because every time they tried to submit a proposal, the documents kept getting overfitted.”
Rudolf de Schipper: I guess the AI finds it funny.
Stefan Morcov – Hermix: Yes, exactly. Here’s another: “Why do public sector sales bring letters to meetings? Because they always want to reach new heights in bureaucracy.” This might be a little offensive to some.
Stefan Morcov – Hermix: I think this is an interesting addition to the tool because often, when replying to an RFP, you come up with impromptu questions. At the beginning, typical questions arise like, “How many CVs do we need?” If you have that question, you can get a quick answer.
Rudolf de Schipper: Even further along, after writing your proposal, you might have questions like, “Are we actually addressing this requirement?” You could ask the AI for clarification on requirements from the RFP.
Rudolf de Schipper: Many participants in this webinar might have faced unexpected questions during approvals. It would be beneficial to ask the AI for answers based on the RFP. For example, if someone asks, “What’s the place of delivery?” it may not always be straightforward if there are multiple locations involved.
Rudolf de Schipper: Quick access to data, as well as interpreted data, is essential. As you said, sifting through 25 annexes isn’t productive. While it may be done with good intentions by the buyer, it’s not always easy to utilize all the information present in an RFP.
Rudolf de Schipper: There are multiple small AI solutions in this ecosystem. We have one that creates summaries and a bot you can interact with. It’s not one massive AI system; it’s a collection of smaller tools. For now, this is how the industry is addressing these challenges.
Rudolf de Schipper: So, maybe we shouldn’t worry too much about AI taking over.
Stefan Morcov – Hermix: I fully support this. I agree that the biggest challenge in public sector sales, and in large, complex proposals, is the significant investment required to write them.
Stefan Morcov – Hermix: For instance, when trying to sell to a big corporation like Vodafone, you invest similarly as you would when writing a 50 million proposal for the European Commission.
Stefan Morcov – Hermix: One major challenge we face is that writing a proposal can require an investment of tens of thousands of euros. A customer I spoke with mentioned they had proposals where they invested €200,000. Writing proposals often involves multiple people working together over several months, which is extremely expensive.
Stefan Morcov – Hermix: Even before that, screening proposals can be a daily task. If you’re in a niche market, you might get 2-3 relevant proposals daily. In a larger market, we screen around 2,000 proposals per day with Hermix. Imagine receiving 5 notices from whatever system you’re using, maybe Hermix or another one. You then need to assess whether you should pursue that tender, which can take 30 minutes to 2 hours to understand the technical requirements.
Stefan Morcov – Hermix: Additionally, understanding the commercial environment and competition can take 2 hours or even 2 days. You want to target tenders with low competition and substantial budgets, following the blue ocean strategy—you want to be the biggest or only shark in an ocean full of tuna.
Stefan Morcov – Hermix: So, how do you find the part of the ocean with significant funds, large budgets, and minimal competition?
Stefan Morcov – Hermix: I was working on a regular tender for about 2 hours, maybe 2 to 3 days for a more important tender to fully understand the context and nuances.
I agree with your points. The costs involved are significant. As for solving these types of problems, there is no silver bullet—no one solution that fixes everything overnight. However, we can integrate various tools, each designed to address specific issues and automate certain processes. This is what we mean by “fit for purpose.”
Regarding data cleanup, I’ll quickly address a question that was raised: how we handle data cleanup and whether we use machine learning or just basic data analysis. The answer is that we use both approaches. Traditional SQL performs many useful functions, and we still rely on it heavily. We also utilize scoring methods, such as Levenshtein distance, alongside more advanced techniques like semantic context summarization and translation.
For instance, our tool can translate requirements, and I’ve tested it successfully with tender documents in Slovene and French, translating them into English. Traditional SQL alone cannot handle this level of complexity. We also conduct graph analysis to understand the relationships between entities, modeling the market as a large graph where nodes represent clients, suppliers, authorities, and contracts. This allows us to detect anomalies and understand partnerships within consortia.
Additionally, we leverage machine learning with our cleaned-up data. We’re collaborating with the University of Luxembourg on an Eureka project, focusing on specific datasets to extract actionable intelligence. So, it’s very much a combination of traditional and modern methods.
As for how partnerships and consortia are displayed, let me demonstrate.
Here’s an analysis of the market, showing contract values and geographic client locations. For example, I’m examining a company in Luxembourg called Anthrop, and you can see their top clients here. We provide partnership analysis that tracks history over the last 8 years, highlighting key partnerships and associated contracts.
We also feature configurable graph displays, allowing you to select depth levels and the number of partners displayed. This helps manage the complexity of the information, ensuring readability.
Now, addressing another question: the tool for analyzing standard documents currently works only for publicly available tenders. Our platform indexes and analyzes these documents four times a day. We are working on adding functionality for users to upload their own documents, but that’s still in the prototype stage.
Most public information about submitted proposals is typically not disclosed, especially for unsuccessful bids. This means we often don’t have access to that data.
Rudolf de Schipper: We’re exploring the future of AI in this domain. Currently, we focus on summarizing RFPs and extracting requirements. The next logical step could be using AI to write RFPs. We’re experimenting with generating coherent text based on our needs.
However, generating impactful text is still a challenge. Clients will likely also employ AI to evaluate our proposals, which could create a cycle where AI drives both proposal writing and evaluation, potentially reducing the focus on quality in favor of automation.
The concern arises: will contracts be awarded based on the quality of AI used in proposal writing, or will it revert to a purely price-based market?
There’s also the question of whether AI can help us develop pricing strategies, a topic worth revisiting in the future as we gain more insights into these technologies.
Stefan Morcov – Hermix: It’s an interesting discussion about the move towards price-only evaluations. We are witnessing this trend in tenders, as exemplified by a recent tender from Digita Suit, which is entirely price-based.
With AI increasingly generating corporate messages on platforms like LinkedIn, we find ourselves in a situation where proposal quality may decline if everyone relies on AI to write them. Historically, long, unwieldy proposals were rarely read, and now there’s a push for conciseness and clarity, aided by technological advancements.
We find ourselves at a crossroads: on one hand, envisioning a future where AI alleviates manual tasks, and on the other, confronting the potential dystopian outcome where automation leads to a purely transactional market devoid of substance.
Stefan Morcov – Hermix: Automatically generated text. They have a set of rules, a few hundred or thousand lines that combine in reports. Accounting is a very clear science. Not all technologies are useful for everything.
Rudolf de Schipper: An interesting remark by Laura Price: this only applies to standard goods and services. I wholeheartedly agree with that.
Stefan Morcov – Hermix: Yes, I agree! Great observation. It makes you think about what the client has in mind when they do a price-only RFP. This likely means they consider what they want to buy as a standard good or service—very procedural, as you mentioned, a bunch of rules and nothing else. For creative and strategic services, price-only evaluations may not lead to quality.
Rudolf de Schipper: The word “creative” struck me. We discussed this yesterday, Stefan: what does “creative” mean when AI generates beautiful pictures? Is it something we would call creative, or is it merely rehashing existing material in a way we’ve never seen before?
Stefan Morcov – Hermix: Absolutely. There are two points to this. First, I fully agree that you can apply pricing and price evaluation to standard goods and services. This is where public procurement balances more towards technical evaluation over price evaluation, especially for creative, strategic, or complex projects. Evaluating prices for non-standard creative or complex environments is challenging.
Secondly, AI is only as good as the humans managing it. I believe artificial intelligence is just a tool; it can be used for good or bad, as with any technology. Even a tractor can be misused.
When discussing creativity and technology, it’s important to note that technology is used as a tool to achieve objectives like winning tenders and having the best proposals.
Stefan Morcov – Hermix: If the tender requirements and proposals are generated by AI, how do you differentiate? I believe differentiation comes from creativity. You need human minds—teams of smart people, technical architects, and visionaries who can generate original ideas and intelligent architectures. Tools can assist in putting these ideas on paper, but the original concepts must come from humans.
There is a common misconception that AI creates solutions. While it may seem creative at times, it’s essentially compiling existing text. We are transitioning from “Let me Google this for you” to “Let me ask ChatGPT this for you.” AI answers many queries accurately, but it compiles existing information rather than creating original content.
Stefan Morcov – Hermix: Regarding graph analysis, it’s a topic I learned in high school and university—calculating distances in nodes, greedy algorithms, and so on. We can assess the distance between two nodes based on contracts signed between partners and their values, leading to scoring.
Stefan Morcov – Hermix: There are many questions coming in. For instance, is AI forbidden by organizations for proposal reductions? I don’t think so. There was a brief attempt to restrict AI in Italy when ChatGPT first emerged, and in New York, public schools banned it for a month. However, in organizations, I have not heard of prohibitions. AI is a useful tool, and we use it daily without realizing it.
Stefan Morcov – Hermix: Technologies like automatic transcriptions and translations in Zoom are forms of AI. We are already employing AI in our daily activities. I do not know of organizations forbidding AI in proposals. I encourage using AI, though I don’t believe it is creative or smart enough on its own to understand context.
Stefan Morcov – Hermix: A comment from Roswan: indeed, the publications office for open tenders is a modern public authority embracing AI for efficient public services. It’s commendable. We learn from their integration of AI, both internally and externally.
Stefan Morcov – Hermix: The private sector tends to move faster for various reasons.
Stefan Morcov – Hermix: We have a few more questions. What is the current position of EU procurement towards AI-generated tenders? Honestly, I don’t know. If anyone has insights, please share. I suspect there is no consensus on this matter.
Rudolf de Schipper: I agree there is no general consensus. From the perspective of an objective buyer, if you can offer the best proposal using AI, go for it. However, a more human-centric buyer might prefer you to sweat over the RFP.
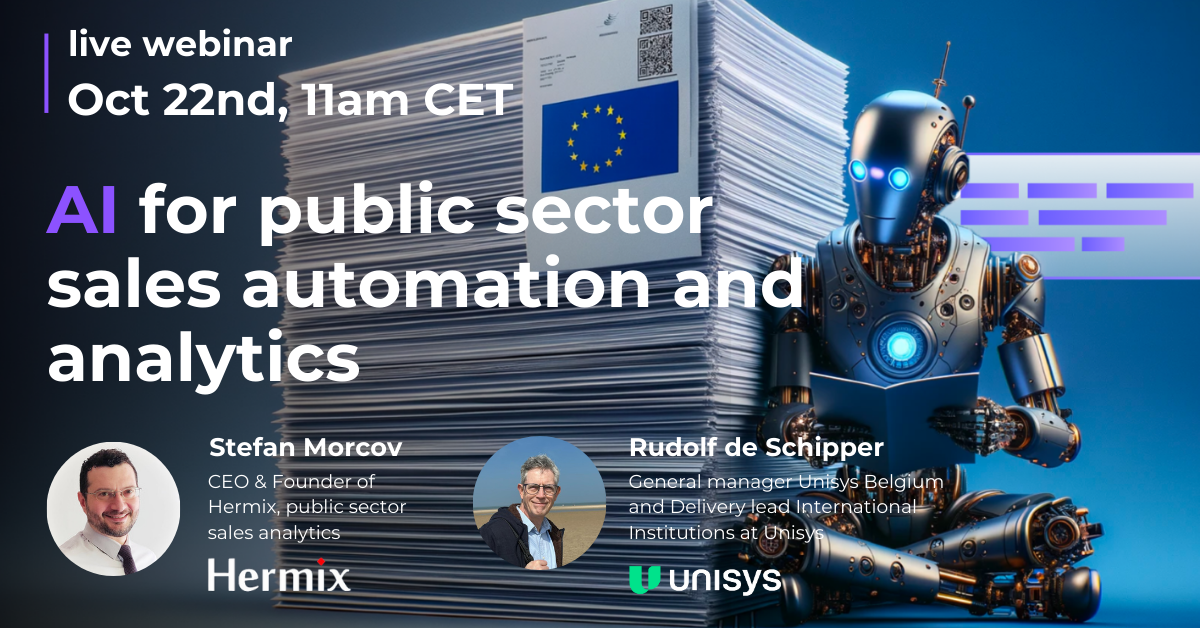